Clinical care accounts for only 10-20% of patients’ health outcomes, while socioeconomic, environmental, and behavioral factors may contribute to the remainder (Hood, Carlyn M., et al., 2016). Recently, the widespread adoption of Electronic Health Records (EHRs) has generated large volumes of clinical data.
This is an enabling resource for developing machine learning (ML) models to study patient outcomes. However, information about social determinants of health (SDoH) is usually recorded in unstructured clinical notes, which hampers access to this information. Thus, most current clinical research using EHRs focuses heavily on clinical factors and consequentially may lead to health inequalities. Despite growing interest in incorporating SDoH into clinical decision-making, these factors are studied in isolation. However, social determinants are often interconnected and should be considered in aggregate to improve health outcomes and reduce disparities. In this research, we leverage a combination of structured and free-text data in EHRs to develop novel natural language processing and ML models to extract nonclinical factors. We use these determinants to develop and validate context-sensitive and individualized polyrisk scores to prioritize high-risk patients using both clinical and interacting social factors. These scores will complement the existing EHR data when developing outcome prediction models and help provide tailored interventions in our health system.
Accepting Students
Health Informatics, Machine Learning, Natural Language Processing, Software Engineering, Information Visualization
Lead Faculty
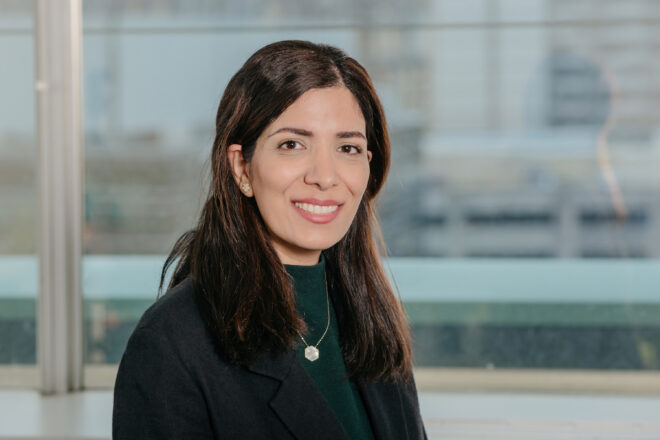