Machine Learning in Health: The Future of Saving Lives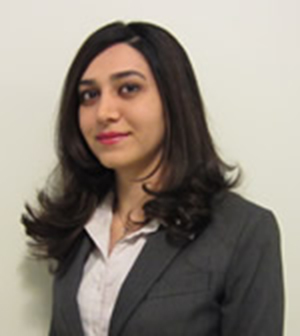
Elham Dolatabadi, PhD, Vector Institute
Machine learning (ML) advances are revolutionizing the healthcare system, from changing the way decision makers think about providing care to optimizing operational challenges across hospitals (e.g. emergency departments and operation rooms). The application of ML in healthcare is a double-edged sword. Health creates some of the most challenging and under-investigated domains for ML research including time series analysis, dealing with missingness and unobserved data, explainability, and transfer learning. The research in the intersection of health and ML therefore, creates a large number of openings for scientific innovation as well as improvement in health outcomes. In this talk, I will provide a general overview of how ML can be applied to health and will be addressing three methodological challenges arising from health data through recent research such as learning a rich representation of heterogenous and multimodal health data, handling unequal time intervals between the observations, and summarizing raw clinical texts.
Elham Dolatabadi is currently a Technical AI staff scientist at Vector Institute. Before joining Vector Institute, she was a Data Scientist at RBC. Prior to her role at RBC, she was a postdoctoral researcher at University Health Network where her research focused on applying ML techniques in the development and evaluation of intelligent health monitoring technologies. She was specifically contributing to enabling new classes of diagnostic tools for healthcare that use ML to retrieve subtle information from everyday activities, as well as large clinical records that provide reliable prediction for future falls risks. Elham finished her PhD in 2015, at the University of Toronto. Her PhD research involved developing Bayesian and probabilistic generative ML approaches for addressing challenges associated with human gait modeling. For her master studies, she was a member of a wireless communication and stochastic process group and her master’s research involved modeling and stochastic analysis of wireless sensor networks for health monitoring.
Approaching the Promise of Artificial Intelligence & Big Data in Healthcare
Health systems, services and policy researchers are increasingly interested in the potential for AI and Big Data to address challenges ranging from complex societal problems to those relating to individual care.
For this reason, the Health Services, Systems & Policy Seminar Series at the Institute of Health Policy, Management and Evaluation (IHPME), University of Toronto will focus this year on the potential and limitations of AI and Big Data to improve healthcare.
Canadian and international researchers and practitioners from an array of disciplines including computer science, decision sciences, predictive analytics, and bioinformatics will join us to discuss applications of AI and big data to issues ranging from genomics, radiology, public health and hospital scheduling to blood transfusion.
Please consider forwarding this information to any colleagues who might be interested.
Related Events
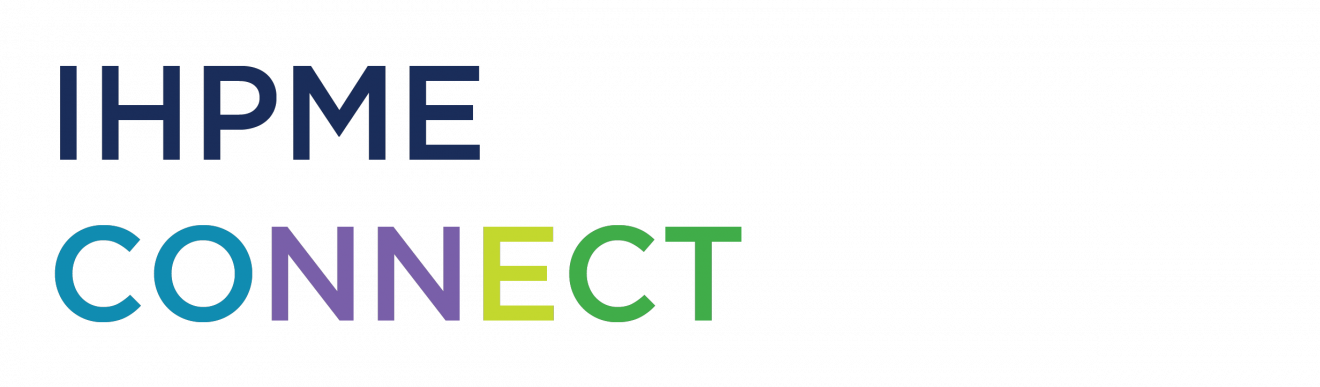
Sign up for IHPME Connect.
Keep up to date with IHPME’s News & Research, Events & Program, Recognition, e-newsletter.
Subscribe to Connect Newsletter
Get in Contact
Communications
Marielle Boutin
Email Address: ihpme.communications@utoronto.ca