Prerequisite
IHPME Students:
HAD5307H Introduction to Applied Biostatistics and HAD5316H Biostatistics II: Advanced Techniques in Applied Regression Methods and at least 2 research methods courses (e.g. HAD5309H, HAD5303H, HAD5306H, HAD5763H, HAD6770H)
Public Health Sciences (PHS) students:
CHL5210H Categorical Data Analysis and CHL5209H Survival Analysis I and CHL5207Y Laboratory in Statistical Design and Analysis (or CHL5208Y Advanced Laboratory in Statistical Design and Analysis)
Or CHL5201H Biostatistics I and CHL5202H Biostatistics II and at least 2 epidemiological methods courses (e.g. CHL5401H and CHL5402H or equivalents)
Objectives
- Develop an understanding of causal reasoning, and state research questions and assumptions in explicitly causal terms, using potential outcomes
- Construct and interpret causal diagrams
- Understand the statistical underpinnings of various methods to control for confounding
- Choose an appropriate method to answer a causal inference question and interpret the results
- Implement/apply the methods in R
Description
This course is an introduction to modern causal inference theory and methods applicable to clinical and epidemiological research. The presentation is mathematically precise, but accessible to students of various disciplines of health sciences with some previous background in biostatistical and epidemiological methods as specified above. This course will introduce students to the potential outcome (counterfactual) model for causation, with brief forays into alternative conceptual models such as causal diagrams, structural equations and target trials. The statistical methods covered in the point treatment setting include propensity score estimation, direct standardization/g-computation, marginal structural models estimated using inverse probability of treatment weighting, doubly robust estimation, instrumental variables and principal stratification. We will also cover extensions to causal inference from longitudinal data subject to time-dependent treatment-confounder feedback, mediation analysis, sensitivity analysis for unmeasured confounding, and use of machine learning methods in causal inference. R statistical environment is used for instruction.
Instructors
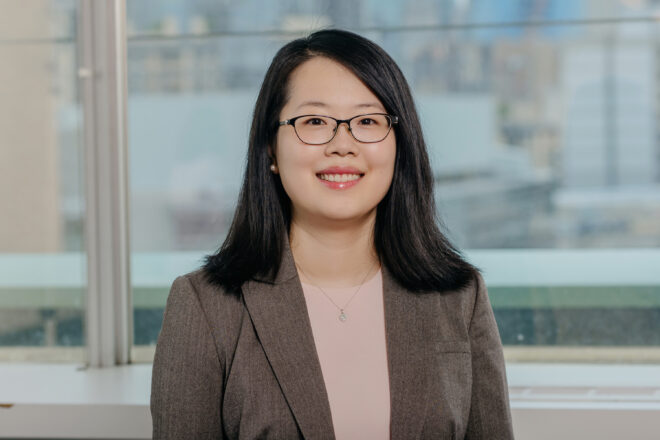
Kuan Liu
Olli Saarela
Evaluation Breakdown
- Participation
- 10%
- Assignments
- 40%
- Presentation
- 10%
- Final Project
- 40%